Machine Learning in Trading: A Singapore Perspective
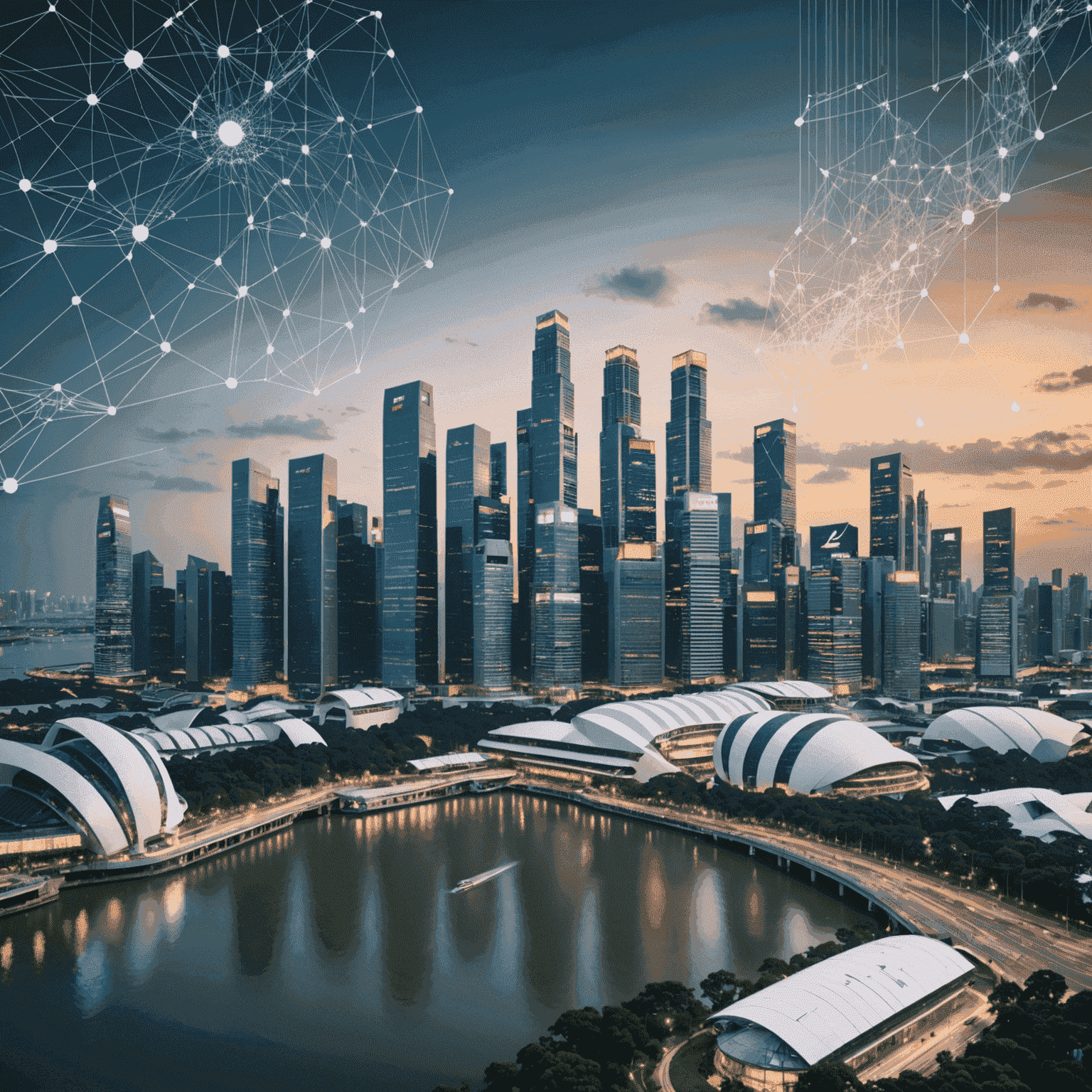
The intersection of machine learning and trading analytics is reshaping Singapore's financial technology landscape, offering unprecedented opportunities for market analysis and decision-making.
The Rise of ML in Singapore's Trading Sector
Singapore, as a global financial hub, has been quick to adopt and integrate machine learning technologies into its trading infrastructure. Financial institutions and fintech startups alike are leveraging these advanced algorithms to gain a competitive edge in the market.
Key Applications of Machine Learning in Trading
- Predictive Analytics: ML models are being used to forecast market trends and asset prices with increasing accuracy.
- Risk Management: Advanced algorithms help in identifying and mitigating potential risks in real-time.
- Algorithmic Trading: ML-powered trading systems execute trades at optimal prices and times, often outperforming human traders.
- Sentiment Analysis: Natural Language Processing (NLP) techniques analyze news and social media to gauge market sentiment.
Singapore's Fintech Innovation
The Monetary Authority of Singapore (MAS) has been instrumental in fostering an environment conducive to fintech innovation. This has led to the emergence of several cutting-edge ML applications in trading:
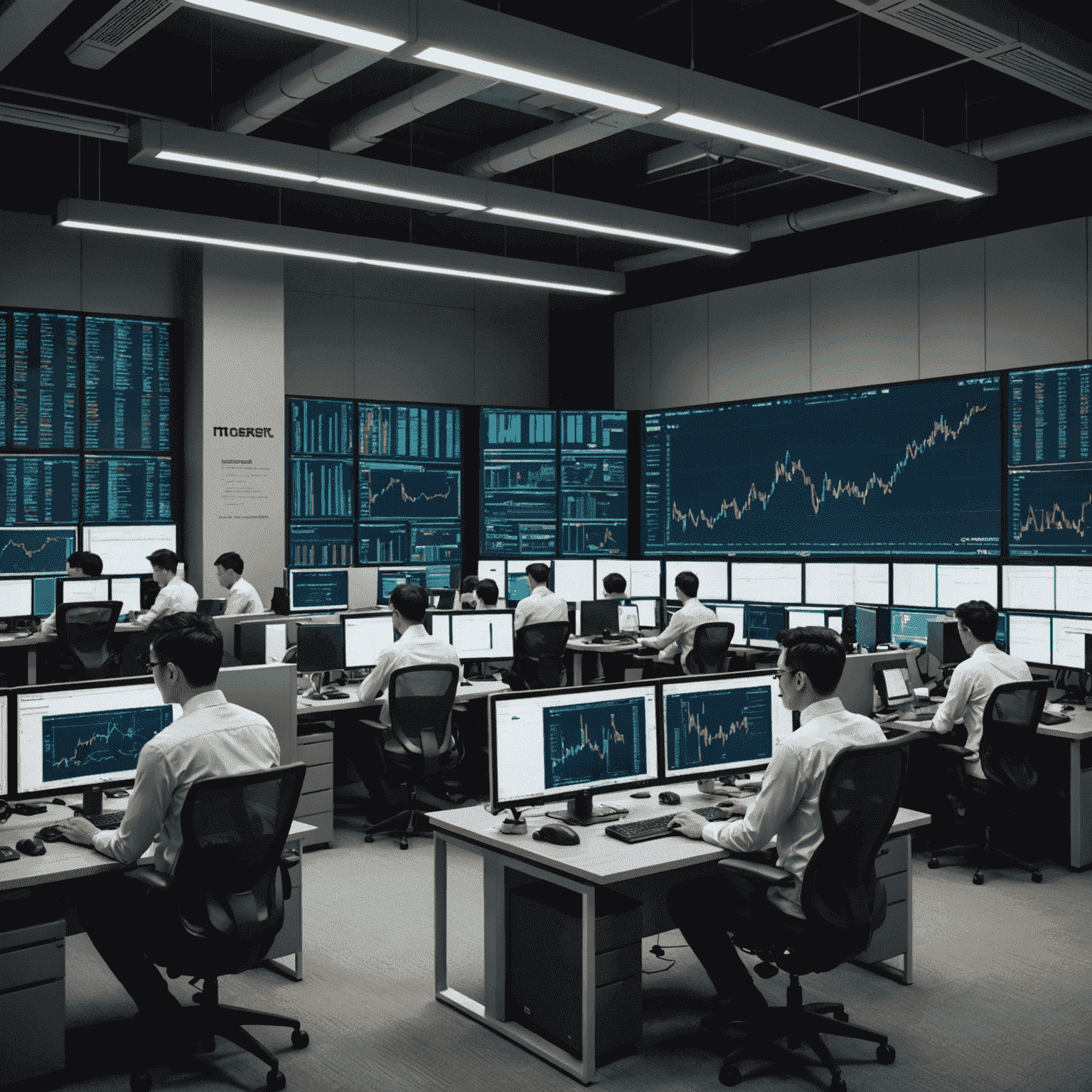
Case Studies
- ATAS (Advanced Trading Analytics System): A locally developed platform that uses ML to provide real-time market insights and trading recommendations.
- Robo-advisors: AI-driven investment management services that are gaining popularity among retail investors in Singapore.
- High-Frequency Trading (HFT) Systems: Sophisticated ML algorithms enabling ultra-fast trade execution in microseconds.
Challenges and Future Outlook
While the adoption of ML in trading has shown promising results, it's not without challenges:
- Data quality and availability
- Regulatory compliance and ethical considerations
- The need for skilled professionals who understand both finance and ML
Despite these challenges, the future of ML in Singapore's trading landscape looks bright. As technologies continue to evolve, we can expect even more sophisticated applications that will further revolutionize the way trading is conducted in the city-state.
Conclusion
Machine Learning is not just a buzzword in Singapore's trading sector; it's a transformative force that's reshaping the industry. As the financial hub continues to embrace and innovate with ML technologies, it solidifies its position as a leader in the global fintech arena. The synergy between cutting-edge technology and financial expertise is paving the way for a new era of trading analytics, promising more efficient, data-driven, and potentially more lucrativeable trading strategies.